“Meta stock skyrockets (probably, you’re welcome Marky Z.) as Elumynt reveals in-platform attribution is under reporting revenue by a degree of up to 81% post iOS14 causing brands to flock back to this channel!”
In a crowded marketing landscape full of buzzwords, acronyms, and best of all memes (which this article will be sure to have) — three recent standouts include: “data-driven”, “multi-touch attribution” or to make it an acronym MTA, and iOS-anything. So, what do these three things have in common? Agencies and ad tech companies love building expensive and often misleading solutions around them to proclaim that they are indeed “data-driven”.
A lot of agencies like to sell themselves to clients by claiming they are top-tier in making “data-driven decisions.'' Maybe they leverage a multi-touch attribution (MTA) tool to stake this claim, maybe they have a fancy spreadsheet spitting out the top-performing Facebook ad based on a nice fluffy KPI-like in-platform reported ROAS (AKA garbage metric). The problem with both of these is that they are at best a 2D (ex. Reported ROAS) or 3D (MTA) “image” of what’s going on forced into a table of numbers upon numbers, and ultimately not very intuitive or actionable.
Because of the recent changes in the data-privacy landscape such as iOS14.5, the deprecation of cookies, etc., these minimally dimensional models are no longer good enough to use for decision-making nor should they qualify as being “data-driven”. Worse, they were never actually good enough in the first place as AdExchanger so eloquently highlighted based on MMA Global’s 2022 State of Attribution report citing “the overall Net Promoter Score of MTA solution providers is…still in the toilet” coming in with a lackluster “minus 16” rating (source: https://www.adexchanger.com/advertiser/more-marketers-are-adopting-multi-touch-attribution-but-theres-still-some-frustration)
So how do we go from this:
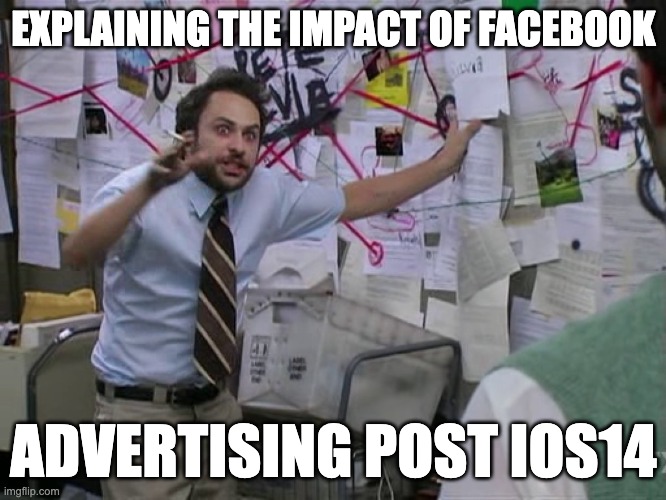
To this?
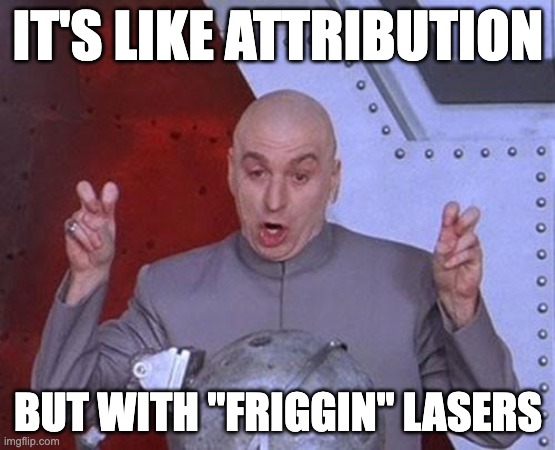
Implement Post-Purchase Surveys
Post-Purchase Surveys are inexpensive and can offer deeper insight into the actual source consumers are valuing as the driver of their purchase decision. To demonstrate the importance of this, take for example our client, who we will refer to as “Client X”. Client X was leveraging Facebook (scaling period-over-period 60%) as a vessel for bringing in new customers with the platform reporting a return of $240K when using a 7-day click, 1-day view attribution window. Google Analytics (GA), for this same time period offered an even bleaker $80K from 1.7k conversions when using source/medium reporting. In consulting the path’s report this figure rises to $90K with a suspicious 3.7k (nearly double last-click reporting) conversions credited to Facebook. Catching our attention even more though is the rise in the revenue credited to source/medium direct/(none) for this same period growing 60% from $225K to $360K in revenue (last-click).

With none of these sources saying even close to the same thing, how are we to trust one over the other? The answer: We don’t.
We know Facebook is limited in what conversions it can take credit for in-platform and anything last-click only is bound to be inaccurate when evaluating a more top-of-funnel tactic like any form of social media. So what did we find when digging into the post-purchase survey data for Client X?
Both Facebook and GA Reporting are Missing the Big Picture
Of the 37,479 survey responses collected by Client X, after ACTUAL purchases, for this timeframe, 6,597 of them cited a Facebook/Instagram Ad as the driver of their purchase. A shocking difference from the 1.7k (last-click) or 3.7k (paths reporting) reported conversions credited to Facebook via Google Analytics. Said a different way, this survey reported that 1 in 5.7 purchases is driven by a Facebook ad (responses for FB/IG Ad divided by total responses). This equates to $168K in sales for Client X (as reported through the survey). Two core elements make this figure so incredibly important: response rate AND UTM parameters collected at the response time.
The response rate (the volume of responses captured out of the total volume of surveys issued) for this period was 45.56%, meaning this data really only represents approximately half of the total purchases that occurred.
If we assume the ratio of those influenced by Facebook/Instagram Ads was even just directionally accurate for ALL purchases, that would mean Facebook was likely responsible for driving $368K in sales (Reported Sales / Response Rate). Now if we look back at the Google Analytics results, that means likely direct was misattributed with potentially $288K in sales that were driven by Facebook (Modeled Survey Revenue $368K minus GA credited last-click revenue $80K). Meaning 78% of the conversions credited there are misattributed to the true source of influence! You can see how we landed on these figures with the visualization below. You can also apply this same logic to in-platform reporting (spoiler alert) to understand what the degree of difference is between what real customers are saying and what the ads platforms are saying.

To help corroborate this thinking, we turn back to the survey data again. For each response collected, so too is a corresponding UTM parameter captured. Of the 6,597 responses for Facebook/Instagram Ad, 98.15% of them were tied to a UTM of direct / (none). Here’s how that breaks down:
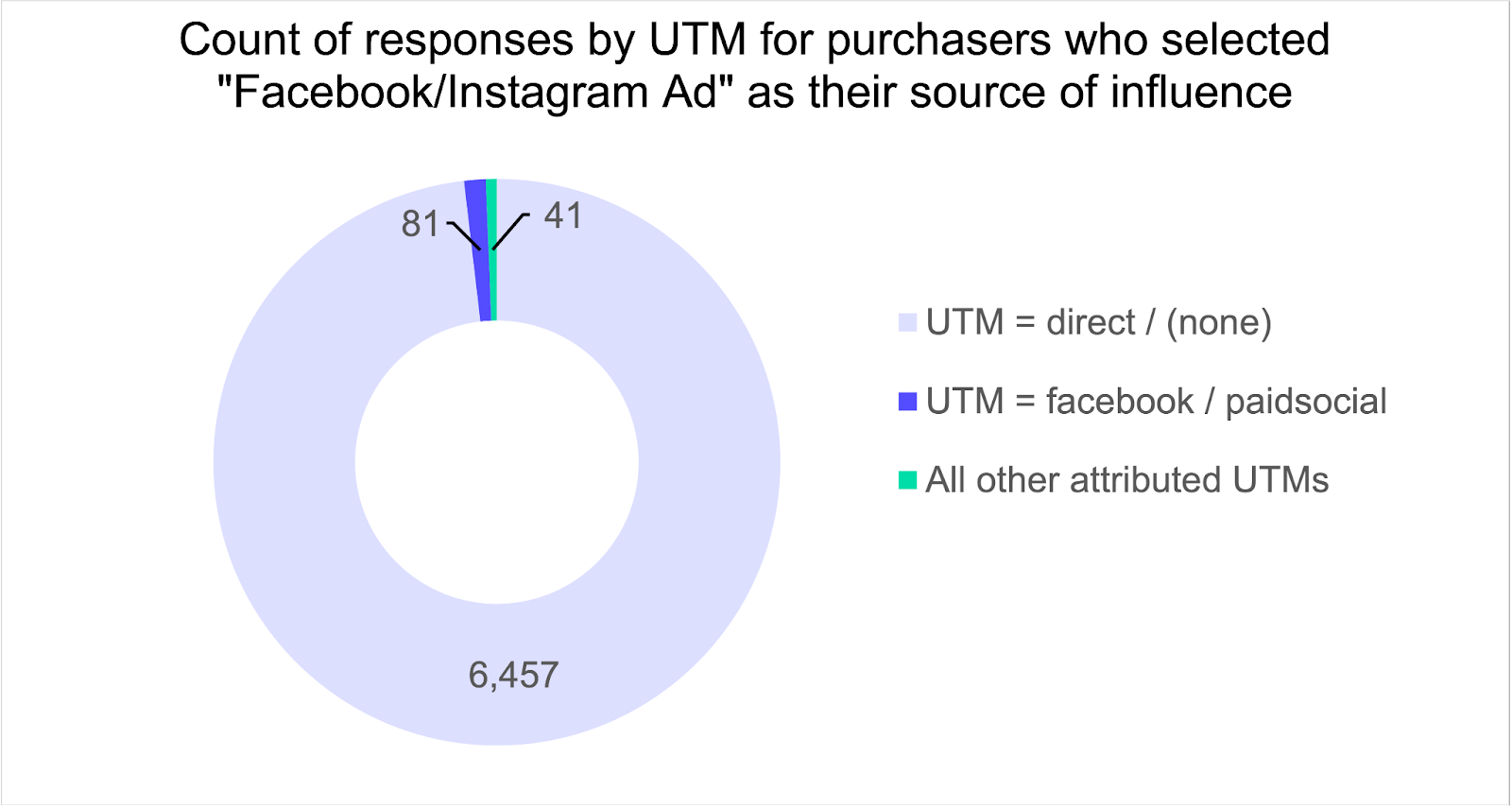
Yeah, our nifty UTM’s got attributed with that tiny sliver of purchases you see above…or maybe you don’t see it because it’s so small…
All this is to say if we go off Facebook-reported revenue or Google Analytics-reported revenue alone - we are likely missing out on the true impact of the channel.
Being data-driven requires dimensionalizing our thinking and sadly the current MTA solutions are still often plagued by the ongoing deprecation of cookies, reliance on UTMs, and measuring click-based data alone for the walled gardens of Facebook and Google.
Layering in this post-purchase survey data can more accurately dimensionalize our thinking at a fraction of the cost.
Creating a Four-Dimensional Performance Model
The first step we need to take, is finding our inner peace and conceding to the fact that current reporting tools are are heavily limited by what they can accurately attribute. As a result, we need to create a model that purposely incorporates that thinking and marries various data sources together to better assess the ACTUAL impact of the most limited channels. This could be channels that by design are more top-of-funnel (ToF) and therefore, less likely to win credit from a last-click UTM or even bottom-of-funnel tactics pushing high cost, considered purchases that require more time than a cookie's lifespan allow for it to get credit.
At its core, we need to evaluate the degree of difference across different attribution windows (in-platform data), relative to last-click and paths reporting from Google Analytics, post-purchase survey data, and finally relative to the actual sales as reported by Shopify to ensure things aren’t overinflated.
Here’s how that looks for Client X:
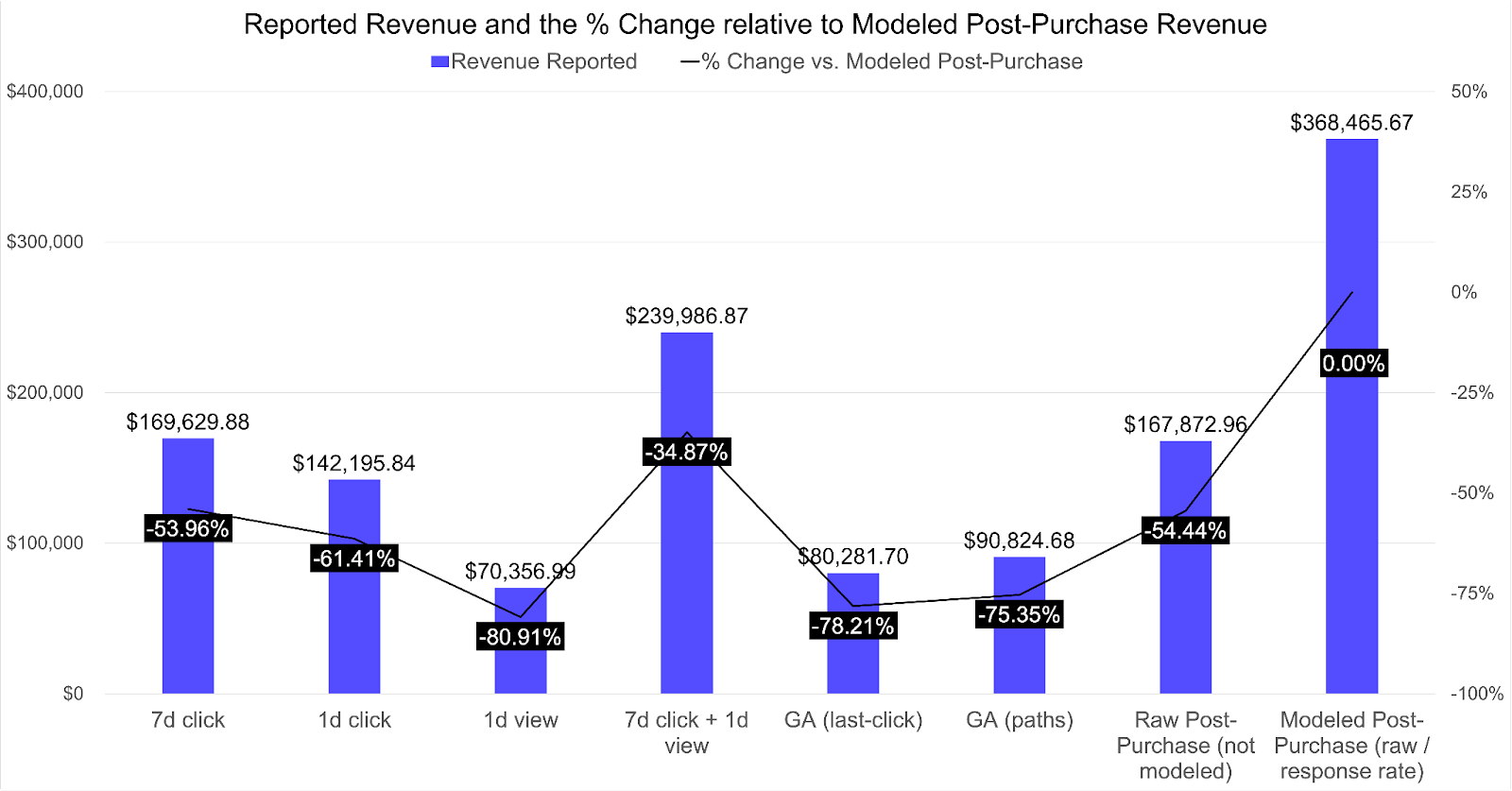
If we were to take the in-platform reporting at face value, we may be actually underreporting the impact of Facebook ads by a margin of nearly 81% if we were using a 1d view attribution window to 35% with a 7d click + 1d view attribution window. Google Analytics is just as big of an offender, ranging a nominal 3% difference between last-click and paths reporting both under crediting Facebook by 75% or more.
If we take the raw post-purchase data, it’s most closely related to a 7d click attribution window for Client X, but that can differ depending on the client and product (again, think about how much ToF you’re doing, how considered the purchase is, etc.). But that at a minimum signals to us that if we want to consult in-platform data for decision making we should use that window to most closely align with the feedback we are getting from actual purchasers. When we model the post-purchase survey data (again, responses divided by response rate to encapsulate a model reflective of 100% of purchases for the given timeframe), it is of course the most generous view by a massive degree. But is it even theoretically possible that Facebook actually drove $368K in sales for this timeframe? The answer: yes. Total Shopify sales for this period for Client X was $3.3M.
Now that we know it’s possible, we can start making more informed decisions. For example, if we were to scale Facebook another 10%, does the volume of responses for the channel increase by a similar degree? Is direct / (none) moving in a way we can correlate with this increased investment based on GA data and the UTM data captured in the post-purchase survey? On and on goes the game of testing hypotheses and either proving or disproving them with this model. While it isn’t necessarily a fancy new dashboard or tool, we can confidently say that the outcomes from this model are far more meaningful and actionable for our clients than something that costs six figures and has a net promoter score that’s in the toilet…
If you remember literally nothing else from this article but this, you’ll be in good shape supporting your ecommerce clients moving forward:
Attribution will never be perfect, so don’t overextend your (or your client’s) budget by paying for the shiny new MTA tool or building the perfect custom dashboard. Guide your decision-making with real-data from actual purchasers in a way that is both cost-effective and actionable by implementing post-purchase surveys.
To sweeten the pot, for those of you that have read this far - our current preferred solution is Fairing (formerly known as Enquire). It integrates seamlessly with Shopify and can be set up in 30-minutes or less. Just be sure to at least sign-on for a plan level (starting at $49/mo) that allows you to “export responses” which is where you’ll be able to dig into all this juicy UTM-level data.
To come full circle, I leave you with this meme - enjoy!
